You are here
Lastest News
-
Dr. Martinez will conduct research at NASA-JPL Robotics
-
New publication in LNNS, volume 965, Springer
-
New graduate with honors
-
Paper by Dr. G. Martinez was the second best paper at ICASAT-2023
-
Dr. Geovanni Martinez gave a talk at ICASAT-2023
Dr. Geovanni Martinez gave a talk at TECCOM 2017 about monocular visual navigation systems
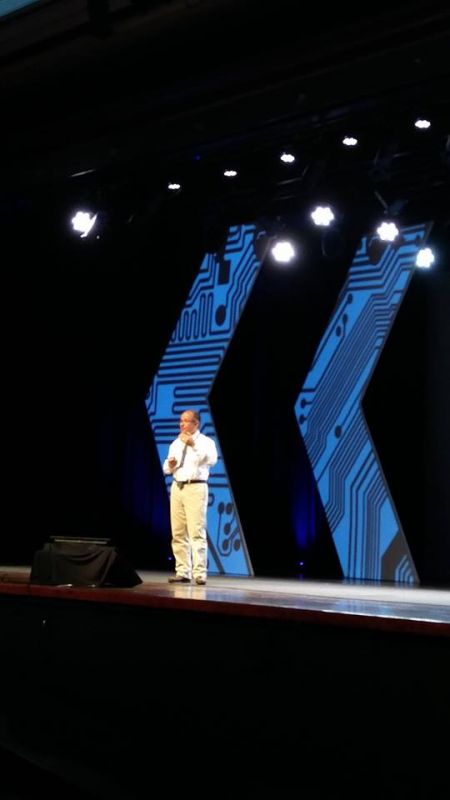
Dr. Geovanni Martinez gave a Talk at TECCOM-2017. In the talk Dr. Martinez explained the Monocular Visual Odometry algorithm based on intensity differences, which has been developed at the IPCV-LAB, and compared it with the Stereoscopic Visual Odometry Algorithm based on Feature Correspondences, which is traditionally used in autonomous robotic and also in palnetary rovers. He described also the experimental results of testing the monocular visual odometry algorithm in a real rover platform Husky A200 over flat terrain for localization in outdoor sunlit conditions.
The Monocular Visual Odometry Algorithm computes the three-dimensional (3D) position of the rover by integrating its motion over time. The motion is directly estimated by maximizing a likelihood function that is the natural logarithm of the conditional probability of intensity differences measured at different observation points between consecutive images. It does not require as an intermediate step to determine the optical flow or establish correspondences. The images are captured by a monocular video camera that has been mounted on the rover looking to one side tilted downwards to the planet's surface. Most of the experiments were conducted under severe global illumination changes. Comparisons with ground truth data have shown an average absolute position error of 0.9% of distance traveled with an average processing time per image of 0.06 seconds.
This is the link to the presentation: